How to get your data ready for generative AI
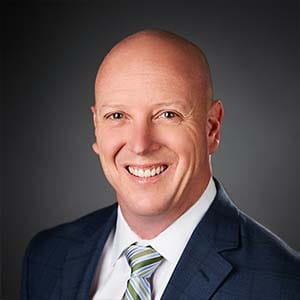
As the world embraces the transformative power of generative AI, organizations are looking for ways to prepare their data to fully leverage the potential of artificial intelligence technology.
Generative AI applications, such as ChatGPT, have the ability to revolutionize various aspects of business operations, from content creation to customer support.
However, to unleash the true power of generative AI, organizations must navigate technical, legal, privacy and strategic challenges related to data management.
Here are the key steps that business leaders should take to optimize their data for successful implementation of generative AI:
Step 1: Enhancing data availability and organization
Improving data availability
To fully utilize generative AI, organizations need to make their data assets readily available and well organized.
This includes uncovering "dark data" – information or content that is often forgotten or stored in offline archives.
By ingesting this untapped data into generative AI applications, organizations can unlock new value streams. It is crucial to make diverse data sources — such as emails, contracts, customer transaction records, text documents, images and legal documents — accessible for generative AI applications to identify patterns and synergies.
Enacting data governance
Establishing data governance is paramount for ensuring the ethical and compliant use of generative AI. Organizations must set up guardrails and controls to determine how and when different types of data can be used by AI applications. This helps mitigate privacy concerns and reduce bias, as AI models tend to inherit biases from the data they are trained on.
Data governance best practices also ensure that the data that is being used by the AI models is accurate and consistent.
Ensuring data quality
The accuracy and reliability of data used in generative AI applications are critical for producing meaningful and trustworthy outputs.
Organizations must prioritize data quality to ensure that the data fed into these AI applications is accurate, complete, timely and consistent. By adhering to the “garbage in, garbage out” principle, you can avoid the propagation of inaccuracies and maintain the integrity of AI-generated content.
Adding data annotations
Generative AI applications rely heavily on metadata to understand and interpret the underlying data.
Organizations should invest in improving their metadata game by providing appropriate data tags, labels, provenance, lineage, quality indicators and other relevant information.
This enables generative AI models to better understand the context and characteristics of the data, enhancing the accuracy and relevance of the generated content.
Curating new data sources
To explore innovative use cases of generative AI, you may need to procure new data sources you don’t currently possess.
This could include social media content, web content, market research data or other datasets offered by data brokers and aggregators.
Establishing a dedicated department for procuring these "data supplies" can help organizations access the necessary data to drive generative AI applications forward.
Validating AI-generated content
As you begin to generate and collect information produced by generative AI applications, you also need to establish policies and procedures to validate the accuracy and reliability of this content.
Generative AI models have been known to produce content that may not always align with factual accuracy.
By implementing additional governance controls and monitoring mechanisms, you can ensure that AI-generated content is appropriately tagged, reviewed and validated before use.
Step 2: Identifying use cases and relevant data sources
To help use generative AI to revolutionize various business functions and processes, start by identifying use cases that align with strategic objectives. Then, use them to prioritize data preparation efforts.
Here are some examples of potential use cases:
Customer service automation
AI language models, such as generative AI, can automate customer service inquiries by answering frequently asked questions, handling simple complaints and resolving issues efficiently.
To implement this use case, you’ll need access to quality customer data, call center records and product information.
Personalized content and recommendations
Generative AI can be used to deliver personalized product recommendations, advertisements and other content to enhance customer experiences.
But to be effective, you’ll need to collect and integrate relevant customer data, feedback and sentiment analysis to gain insights into customer preferences and tailor content accordingly.
Generative AI can assist in content creation by generating marketing and sales content, social media posts, blogs and more.
To leverage this use case, organizations need to curate diverse data sources, such as market research data, competitive insights and industry trends.
Fraud detection and compliance monitoring
Generative AI can play a crucial role in detecting fraudulent activities and monitoring compliance.
You can use generative AI to analyze vast amounts of data and identify patterns that indicate potential fraud or noncompliance.
To implement this use case, organizations need access to relevant transactional data, compliance guidelines and industry regulations.
Training and decision making
Generative AI can be used for employee and customer training, as well as strategic and operational decision making.
Your leaders can leverage generative AI to simulate scenarios, generate training materials and provide valuable insights for decision makers.
To implement this use case, organizations need access to relevant data related to training procedures, historical decision making processes and business objectives.
Step 3: Balancing data security and accessibility
It is crucial to strike a balance between data security and accessibility.
Encryption, access controls and regular data backups are essential for ensuring data security.
At the same time, data should be stored in a centralized location that facilitates easy accessibility. Cloud-based storage and data management tools can provide additional flexibility and scalability for managing data in the context of generative AI.
Understanding the legal implications of generative AI is also critical.
Organizations should be aware of the terms and conditions of AI models, such as OpenAI's ChatGPT.
Clear guidelines should be established to protect sensitive information, comply with privacy regulations and address potential ethical issues that may arise from AI-generated content.
Step 4: Developing a comprehensive data strategy
To fully capitalize on the potential of generative AI, organizations must develop a comprehensive data strategy that goes beyond traditional data management practices.
Here are some key considerations:
Clear objectives and use cases
Identify clear objectives, goals and use cases for leveraging generative AI in line with business priorities. Determine how generative AI can be used to drive cost savings, improve customer experiences, enhance decision making and explore new revenue streams.
Relevant data collection and integration
Ensure that your organization is collecting and making available the right type of data for generative AI applications. This may involve integrating existing databases, leveraging APIs and exploring new data sources to align with the specific use cases identified.
Infrastructure readiness
Assess your organization's existing infrastructure and technology systems to ensure it’s capable of supporting generative AI initiatives. This may require updates to databases, APIs and other IT infrastructure components to facilitate seamless integration with generative AI applications.
Skill development
Invest in upskilling employees to leverage generative AI effectively.
Identify the necessary skills and competencies required to work with generative AI and provide training and development opportunities to equip employees with the required knowledge.
Measurement and evaluation
Define relevant metrics and key performance indicators (KPIs) to measure the success and impact of generative AI initiatives.
This could include metrics related to customer retention, cost savings, risk management, customer satisfaction and new revenue streams. Regularly evaluate the performance of generative AI applications and adjust strategies accordingly.
Generative AI is a game changer that has the potential to revolutionize industries.
By preparing and optimizing data for generative AI, organizations can unlock new opportunities for innovation, productivity gains and revenue growth. As leaders embark on this transformative journey, it is crucial to address the technical, legal, privacy and strategic challenges associated with data management.
By following these steps and developing a comprehensive data strategy, organizations can position themselves for success in the generative AI era.
How Wipfli can help
Wipfli team of technologists can help you with both AI strategy and data integration. To learn more, see our data and analytics web page.