CECL Methodologies Series: Probability of Default
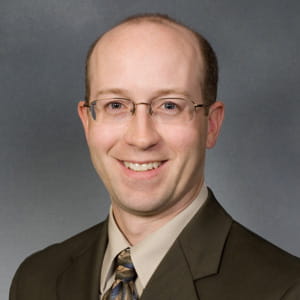
This article is the fifth in our series of articles focusing on the different Current Expected Credit Loss (CECL) methodologies and their pros and cons. Previously, we have looked at the following methodologies:
- Cumulative loss rate (also known as “Snapshot”)
- Vintage loss rate
- Migration analysis
- Remaining life
In this article, we will look at the probability of default method.
Overview
The probability of default methodology is a relatively simple methodology in concept since it only requires three inputs to estimate lifetime losses. The challenging part of the methodology is calculating each of these inputs.
How it Works
As discussed, an institution must calculate three variables in a probability of default methodology:
- Probability of default (PD). First, an institution needs to define what a default is. Generally, this will be a past-due cutoff, for example, 90 days past due. Then the institution must calculate the probability a loan in the pool defaults. This will be initially based on historical performance of the pool and may be adjusted for current and/or forecasted changes in the pool.
- Loss given default rate (LGD). Using historical information about loans that defaulted, the institution must determine the expected loss rate if a loan defaults. Like the probability of default, the institution may directly adjust the loss given default rate for current and/or forecasted changes.
- Exposure (E). Finally, the institution must estimate what the principal balance of the loan will be when a loan defaults.
Estimating each of these variables will take the most work and may require some statistical analysis of historical information. Once these variables are determined, the expected lifetime loss is simply the result of multiplying them together: PD x LGD x E. For instance, let’s assume the following inputs:
- PD = 5%
- LGD = 30%
- E = $10 million
In this example, the estimated CECL loss is 5% x 30% x $10 million = $150,000.
Ideally, each of these three variables will be adjusted for current and forecasted changes individually. For example, based on historical cycles, an institution may find that a 1% increase in regional unemployment may result in a 3% increase in the probability of default. If the institution is forecasting a 0.5% increase in unemployment for the next year, the PD variable in the previous example could be adjusted to 6.5%.
Another example might be that an institution determines the loss given default rate increases by 3% for every 2% decline in collateral prices. If the institution expects collateral prices to decline by 10% in the next year, the LGD input would be adjusted to 45%. These qualitative adjustments for current and forecasted changes will have to be supported in the same way the three inputs to the methodology are supported.
Pros and Cons
The key advantage of probability of default over other methodologies is that it is usually more precise since it relies on more quantitative information. Even qualitative factors for current and forecasted changes are typically based on historical data, and these qualitative factors can be reflected directly in the model as previously noted rather than being “added on” to the quantitative part as in other methodologies. All of this reduces reliance on more subjective factors and should result in a smaller CECL allowance for credit losses than other methodologies.
However, with this added precision comes added work. The institution will need more data to be able to accurately estimate the three inputs to the model. Additional data will be needed to determine whether and how economic factors affect the variables so they can be adjusted for current and forecasted changes. And all of these calculations will probably require some statistical analysis, which will likely require specialized software.
Pros |
Cons |
Very precise methodology that will usually result in a lower CECL allowance for credit losses |
Will require more data to calculate the key variables and the qualitative adjustments |
Qualitative factors can be directly applied to the methodology’s inputs, further “fine tuning” the resulting estimate |
These calculations will probably require statistical analysis to accurately determine the key inputs, which will likely require specialized software |
Concluding Thoughts
The probability of default methodology will generally provide a lower CECL allowance for credit losses over other methodologies because it uses more quantitative information and relies less on subjective analysis. This comes with the cost of having to accumulate more data and utilizing statistical analysis to calculate the key inputs and qualitative adjustments in the methodology. However, the cost of utilizing probability of default may be recouped by being able to utilize additional capital not tied up in a CECL allowance for credit losses.
We will look at the last CECL methodology—discounted cash flow method—in a future article, but if you would like to discuss any or all of the available methodologies in more detail at any time, please contact Brett Schwantes or your Wipfli relationship executive, and we will be happy to set up an appointment with you!
For more information on CECL, please check out our recent articles: